NC Universities Bringing Artificial Intelligence to Manufacturing
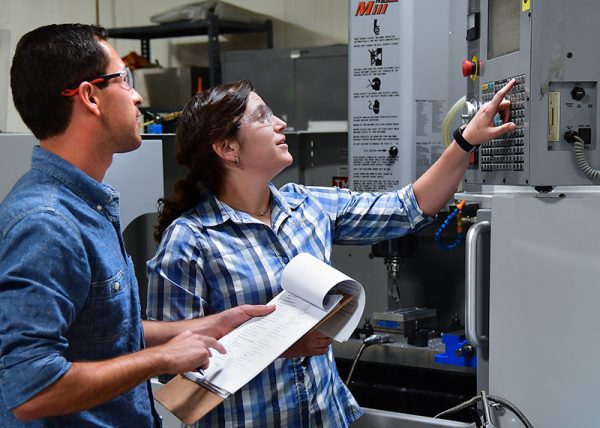
Traditional manufacturing is an important element of North Carolina’s economy, and keeping the state at the forefront of manufacturing technology is vital. Being at the forefront means bringing digital technology to the manufacturing floor, which means artificial intelligence (AI). To develop and advance North Carolina’s use of AI in manufacturing, the state has established a consortium of researchers from UNC Charlotte, North Carolina State University and Fayetteville State University.
The North Carolina Consortium for Self-Aware Machining and Metrology (CSAM) is supported by a $1.66 million Research Opportunity Grant from the University of North Carolina System. CSAM’s goal is to generate new knowledge in the application of artificial intelligence to high-precision part manufacturing and measurement. Specifically, the team is working to develop self-aware operations, or the ability of a production or measuring machine to understand its current state and surroundings and to respond accordingly.
“We are in first year of the four-year project,” said Dr. Tony Schmitz, UNC Charlotte professor of Mechanical Engineering and principal investigator for CSAM. “We are looking for ways to connect artificial intelligence and manufacturing, so we have brought together experts from both fields.”
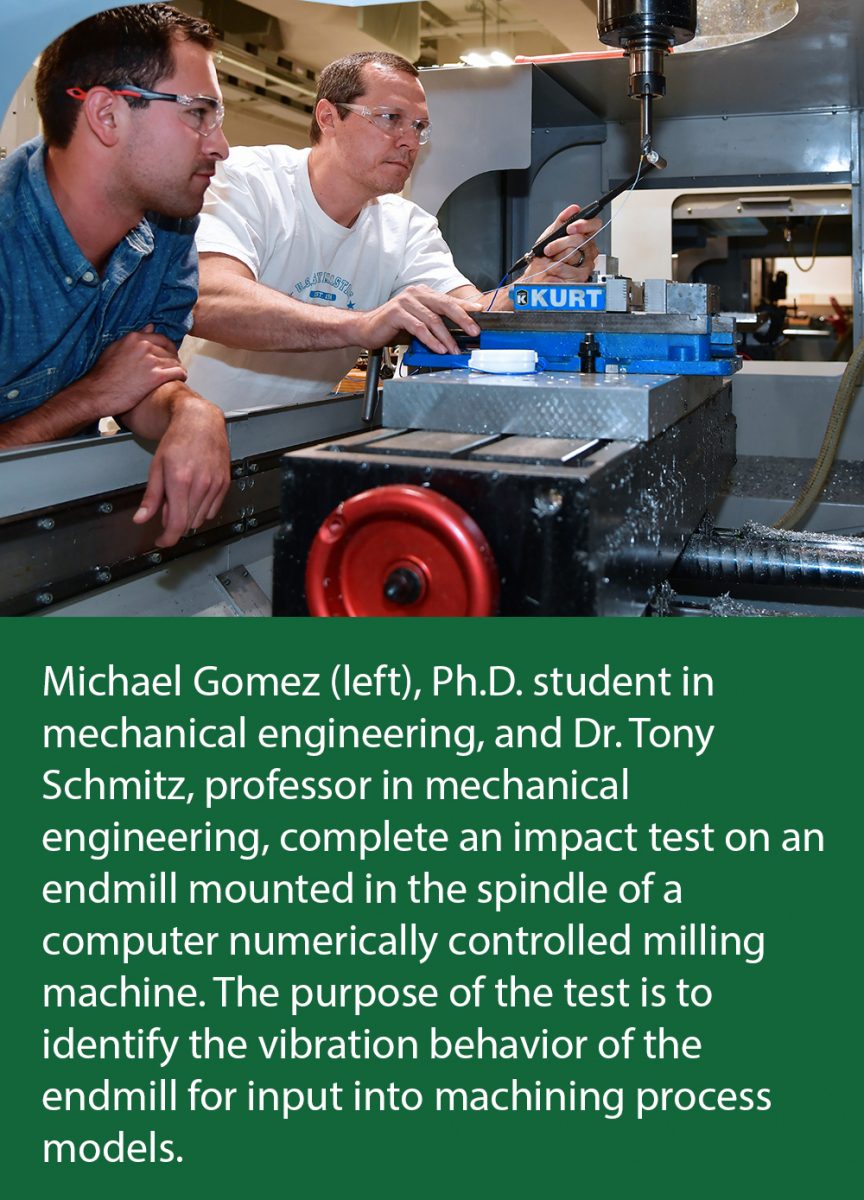
The team’s expertise includes researchers in AI, machining process modeling, measurement science, design and ultra-precision machining of freeform optics, and vision systems. UNC Charlotte’s Center for Precision Metrology is generating the process and measurement data about machining variables and results. This data is then integrated into physics-based process models. North Carolina State and Fayetteville State then couple the physics-based models with data learning algorithms in new hybrid AI modeling approaches.
“Using algorithms in machining isn’t new,” Dr. Schmitz said. “What is new is the amount of data and computer processing power we can apply. Sensors are less expensive now and data is easier to store, so this greatly expands the amount of information we can collect and process.”
CSAM’s first projects include using AI to push the boundaries of ultra-precision diamond turning to produce mirror surface finishes, and developing machining models that enable efficient, stable cutting with no chatter or uncontrolled vibration. The data being collected during the machining processes includes material properties of the workpieces, cutting tool spindle speed, depth of cut, cutting edge geometry, structural dynamics, applied cutting force, and feed rate of the tool past the workpiece. These data are input into models to predict the parameters that will produce the highest quality parts, using metrics such as geometric accuracy, machining time and surface finish.
“By using artificial intelligence we are developing better models,” Dr. Schmitz said. “In the most basic sense, AI is the ability of a computer program or machine to think and learn much like a human being. First, we train the computer model with data, which assigns values to all the connections. Once the model is trained, we can compare its predictions to the actual machining results observed. We keep collecting data during the machining process and continue to update and improve the model. AI helps tell us how to do the machining right the first time.”
A professor of Computer Science at Fayetteville State, Dr. Sambit Bhattacharya specializes in applying AI and computer vision to various applications such as bio-imaging and robotics. He and his research team are now working as part of CSAM to apply AI and machine learning to manufacturing.
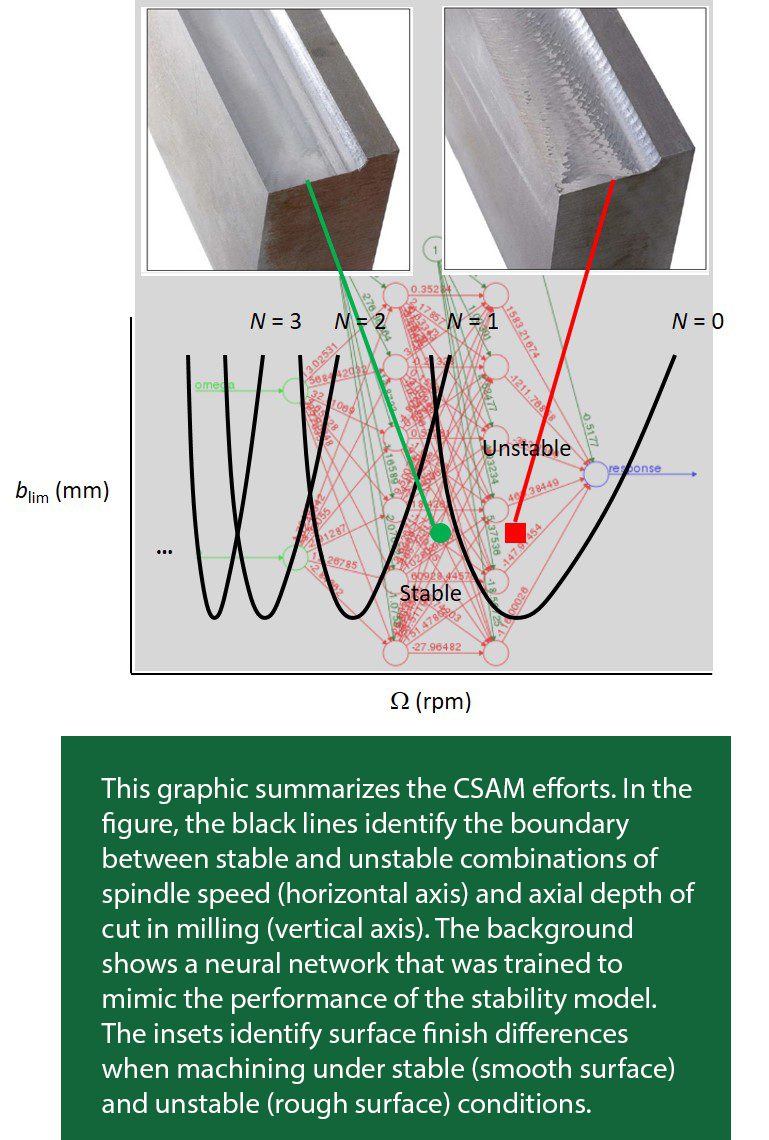
“With traditional AI, we create rule-based algorithms that we apply to data sets,” Dr. Bhattacharya said. “With machine learning, the data is used to train algorithms. We replace the analytical approach by collecting the data, labeling it, and then letting the labeled data train the algorithm. The trained algorithm is then able to make predictions. This is machine learning.”
A relatively new subset of machine learning algorithms is deep learning. Deep learning is able to automatically learn the features of data and typically employs more complex models.
“In this research to solve problems in manufacturing, we are also bringing in deep learning,” Dr. Bhattacharya said. “We learn from the data, but constrain the learning with theory-guided methods. We have reason to believe that this hybrid approach may result in better performance than a purely data-driven approach.”
Also providing her expertise to the CSAM project is Dr. Noel Greis, a research professor at the Poole College of Management at North Carolina State. Her current research focuses on the use of digital technologies and data science tools such as machine learning and AI to build “smart” processes for manufacturing and supply chain management.
“An entirely new language is developing around digitalization with terms like big data, Industrial Internet of Things, smart machining, and the connected factory,” Dr. Greis said. “The challenge for CSAM is to gather all that data, fuse it together, and interpret it for decision-making in manufacturing. Our work is pushing the envelope forward in controlling manufacturing processes.”
Machine learning helps recognize patterns in data and then make predictions, Dr. Greis said. “It’s all about prediction. We need to be able to predict the parameters that will keep machines running optimally. If machines aren’t running optimally, if cutting tools are getting duller faster, or if things are breaking, we want to know why.”
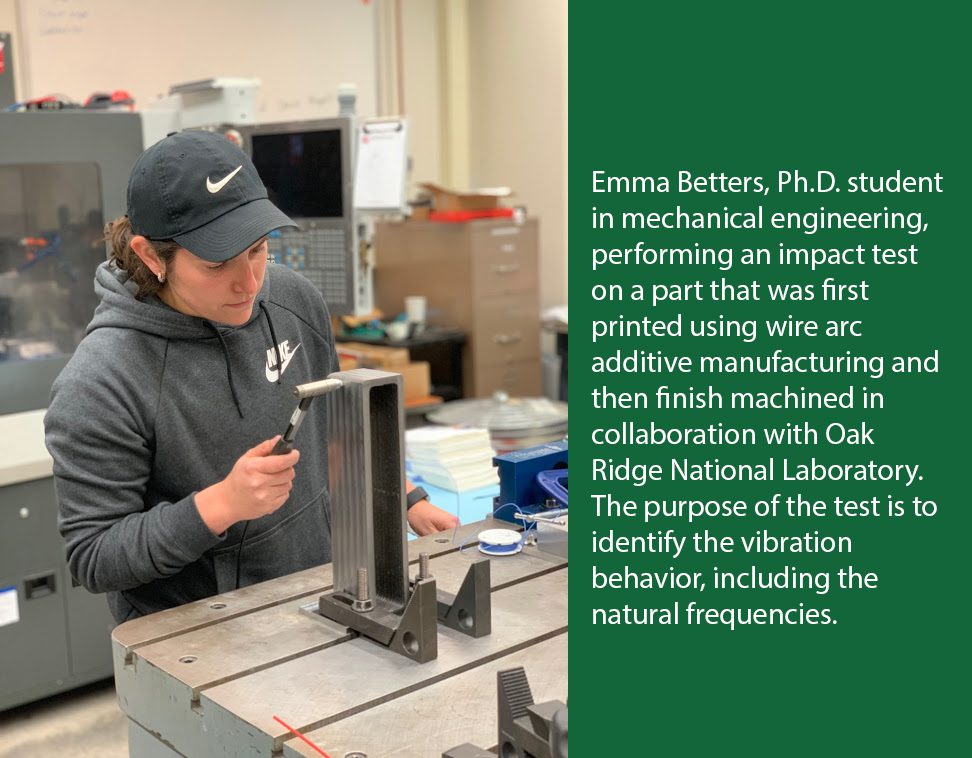
To make better predictions, the researchers are applying physical theory to machine learning. “In manufacturing, we don’t have the huge data sets that we do in other disciplines like computer vision,” Dr. Greis said. “So the question becomes, can we bring theory into the equation to make up for the lack of data? Theory-guided data learning means taking the physical theory that we have about machining and using that knowledge to complement the data. Bringing in theory is one of the most exciting parts of the project.”
Since CSAM started working on its first two projects in September, the researchers have learned a lot about what models are appropriate for manufacturing. “Everyone is very excited,” Dr. Greis said. “Our goal is to advance machine learning for industrial machining applications. And, if we’re successful, manufacturing across North Carolina will benefit.”
Dr. Bhattacharya agreed about the potential gains of the project. “This research can be groundbreaking and transformative for the state,” he said. “In manufacturing there are many problems that can be solved by the data-driven approach. I’m very excited about this project. It is a great opportunity for me and my students, and we’re looking forward to the next several years.”
The CSAM team is working very well together, Dr. Schmitz said. “I am thrilled with this team. So far, this has been one of best experiences in my 18 years of academic research. All of us have gotten out of our comfort zones, learned things outside of our own disciplines, and become students ourselves again.”
CSAM Team Members
- UNC Charlotte – Dr. Harish Cherukuri, Dr. Matt Davies, Dr. Ed Morse, Dr. Tony Schmitz, Dr. Tom Suleski, Dr. Joshua Tarbutton
- Fayetteville State – Dr. Sambit Bhattacharya
- NC State Poole College of Management – Dr. Noel Greis, Dr. Monica Nogueira
- Oak Ridge National Laboratory – Dr. Scott Smith
Industry Partners
CSAM is working in cooperation and sharing information with a number of industry partners including: ATI Specialty Materials, AURA Technology, Blaser Swisslube, Carl Zeiss Industrial Metrology, Caterpillar, DP Technology Corp., Exactech, FLIR Optical Components Group, GE Global Research Center, GE Aviation (Asheville, West Jefferson, and Wilmington), GOM Americas, Intel Corporation, Manufacturing Laboratories, Inc., National Institute of Standards and Technology (NIST), North Carolina Department of Commerce Office of Science, Technology & Innovation, Okuma America Corp., OptiPro, Opto Alignment, OptoMill Solutions, PolylmerPlus, Praemo, Siemens PLM Software, Inc., Structure Medical, and Third Wave Systems